Pre-processing and Handling Unbalanced Data in CNN for Improving Automated Detection of COVID-19 Cases: Preliminary Results
Abstract:
In the context of the COVID-19 pandemic, early diagnosis and treatment are crucial to avoid a drastic increase in the number of new infections. Chest imaging plays an important role in the early detection of the disease since it can be used to identify the initial phase lung infection caused by the SARS-CoV-2 virus. Recently, some researchers have begun to explore Convolutional Neural Networks (CNNs) to detect COVID-19 cases from chest X-ray images. CNN is a category of deep artificial neural networks that has demonstrated great success in computer vision applications, such as video and image analysis. However, this type of network is still affected by abnormal real-world factors such as unbalanced data, presence of noise, blurring, or other quality degradation that can diminish their overall performance when they are not properly handled. This work introduces a methodology to explore and compare the overall accuracy achieved by an Xception-based CNN model when it is combined with benchmark techniques such as unsharp masking, batch balance, and data augmentation aiming at enhancing image details (such as the bone structure and lung) and handling unbalanced datasets. Experiments are done referring to the COVIDx dataset. Preliminary results demonstrate that the proposed methodology leads to higher accuracy when implementing both image enhancement and batch balancing.
Año de publicación:
2020
Keywords:
- Convolutional Neural Networks (CNNs)
- Chest X-ray classification
- IMAGE PROCESSING
Fuente:
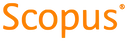
Tipo de documento:
Conference Object
Estado:
Acceso restringido
Áreas de conocimiento:
- Aprendizaje automático
- Infección
- Ciencias de la computación
Áreas temáticas de Dewey:
- Ciencias de la computación
- Medicina y salud
- Enfermedades

Objetivos de Desarrollo Sostenible:
- ODS 3: Salud y bienestar
- ODS 17: Alianzas para lograr los objetivos
- ODS 9: Industria, innovación e infraestructura
