Pbkp_redicting High School Students' Academic Performance: A Comparative Study of Supervised Machine Learning Techniques
Abstract:
The proliferation of mobile devices and the rapid development of information and communication technologies have revolutionized education. Educational data has evolved to be voluminously massive, broadly various, and produced at high velocity. Therefore, computerized techniques for integrating, processing, and transforming data into valuable knowledge have become necessary to improve internal academic processes. Specifically, educational data mining is an emerging discipline concerned with analyzing the massive amounts of academic data generated and stored by educational institutions. In this sense, machine learning algorithms aid decision-makers who are establishing strategies to improve students' learning experience and institutional effectiveness by revealing hidden patterns in academic performance. Thus, this paper describes our comparative study of machine learning techniques to pbkp_redict academic performance. We selected the features that best fit the discovery of patterns in the academic performance of high school students, resulting in a balance between accuracy and interpretability. We implemented six supervised learning algorithms for pattern recognition: Light Gradient Boosting Machine, Gradient Boosting, AdaBoost, Logistic Regression, Random Forest, and K-nearest Neighbors. The experimental results showed that the Gradient Boosting (Gbc) algorithm achieved the highest accuracy (96.77%), superior to other classification techniques considered.
Año de publicación:
2021
Keywords:
- Supervised learning
- Educational Data Mining
- High school education
- Educational Innovation
- ACADEMIC PERFORMANCE
- classification
Fuente:
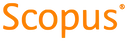
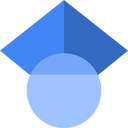
Tipo de documento:
Conference Object
Estado:
Acceso restringido
Áreas de conocimiento:
- Aprendizaje automático
- Análisis de datos
Áreas temáticas:
- Ciencias de la computación
- Ingeniería y operaciones afines
- Escuelas y sus actividades; educación especial