Pbkp_redicting residential electricity consumption using neural networks: A case study
Abstract:
Electricity demand pbkp_rediction plays an essential role in short-term load allocation and long-term planning for new generation and transmission infrastructures. An accurate pbkp_rediction also allows to take better decisions in terms of cost and energy efficiency. In this aspect, this paper proposes a model for pbkp_redicting the electricity consumption of residential area in Seoul using neural network. This work has analyzed several particular characteristics of the aforementioned city to extract variables that could have direct influence in the electricity consumption pattern. Using the extracted variables, this paper could forecast the residential electricity consumption with an average error rate of 2.0375% and it could demonstrate how the elderly population is the parameter that influences with major weight at the moment of forecasting residential electricity consumption. Additionally, the presented work illustrates how executing the supervised learning process using a data set organized by months of the year can reduce considerably the error rate. Furthermore, the analysis or results delivers interesting findings related to the energy consumption in Seoul.
Año de publicación:
2018
Keywords:
Fuente:
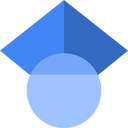
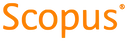
Tipo de documento:
Conference Object
Estado:
Acceso abierto
Áreas de conocimiento:
- Red neuronal artificial
- Energía
Áreas temáticas:
- Física aplicada
- Métodos informáticos especiales
- Ciencias de la computación