Pbkp_redicting software maintainability using ensemble techniques and stacked generalization
Abstract:
The pbkp_rediction of software maintainability has emerged as an important research topic to address industry expectations for reducing costs, in particular maintenance costs. In the last decades, many studies have used single techniques to pbkp_redict software maintainability but there is no agreement as to which technique can achieve the best pbkp_rediction. Ensemble techniques, which combine two or more techniques, have been investigated in recent years. This study investigates ensemble techniques (homogeneous as well as heterogeneous) for pbkp_redicting maintainability in terms of line code changes. To this end, well-known homogeneous ensembles such as Bagging, Boosting, Extra Trees, Gradient Boosting, and Random Forest are investigated first. Then the stacked generalization method is used to construct heterogeneous ensembles by combining the most accurate ones per dataset. The empirical results suggest that Gradient Boosting and Extra Trees are the best ensembles for all datasets, since they ranked first and second, respectively. Moreover, the findings of the evaluation of heterogeneous ensembles constructed using stacked generalization showed that they gave better pbkp_rediction accuracy compared to all homogeneous ensembles.
Año de publicación:
2020
Keywords:
- Homogeneous
- Stacked generalization
- Machine learning
- ensemble techniques
- Software maintainability pbkp_rediction
- Heterogeneous
- Stacking
Fuente:
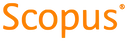
Tipo de documento:
Conference Object
Estado:
Acceso restringido
Áreas de conocimiento:
- Ingeniería de software
- Software
Áreas temáticas:
- Programación informática, programas, datos, seguridad
- Métodos informáticos especiales
- Funcionamiento de bibliotecas y archivos