Pbkp_rediction of CO and HC emissions in Otto motors through neural networks
Abstract:
This paper explains the application of artificial neural networks (ANN) for the pbkp_rediction of pollutant emissions generated by mechanical failures in ignition engines, from which the percentage of CO (% carbon monoxide) and the particulate in parts per millions of HC (ppm of unburned hydrocarbons) can be quantified, through the study of the Otto cycle intake phase, which is recorded through the physical implementation of a Manifold Absolute Pressure (MAP) sensor. A rigorous protocol of sampling and further statistical analysis is applied. The selection and reduction of attributes of the MAP sensor signal is made based on the greater contribution of information and significant difference with the application of three statistical methods (ANOVA, correlation matrix and Random Forest), from which a database that enables training two backpropagation feedforward neural networks, with which a classification error of 5.4061e−09 and 9.7587e−05 for CO and HC, respectively, can be obtained.
Año de publicación:
2020
Keywords:
- carbon monoxide (CO)
- pollutant emissions
- pbkp_rediction
- Neural networks
- non-combustion hydrocarbons (HC)
- DIAGNOSTICS
Fuente:
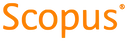
Tipo de documento:
Article
Estado:
Acceso abierto
Áreas de conocimiento:
- Simulación por computadora
- Algoritmo
- Red neuronal artificial
Áreas temáticas:
- Otras ramas de la ingeniería
- Física aplicada
- Métodos informáticos especiales