Prediction of Corrosion of Oil Pipelines in Ecuador based on Machine Learning
Abstract:
The corrosion in oil pipelines not only causes economic losses to Ecuador, but also has harmful effects on both people's health and the environment. This study applied Machine Learning algorithms to predict the level of corrosion based on data collected regarding production and the chemical processes in the oil pipelines. Support Vector Machine (SVM), Random Forest (RF) and XGBoost classification models were implemented and evaluated according to a set of metrics, such as accuracy, precision, and f1-measure, and based on these results a model was selected to tune the hyperparameters to improve the performance of it. In addition, a feature engineering stage was considered, which includes the normalization of the data, the elimination of variables with high correlation and the balance of the data set. 10-fold-cross-validation was also performed. In this case, the results of the analysis showed that the RF model has better performance results than the other models, for instance, RF accuracy was about 20% better than SVM and 5% better than XGBoost, so RF model was selected for the hyperparameters tuning. Besides, a dashboard web application was developed so the end user can make the prediction in a friendly way.
Año de publicación:
2022
Keywords:
- accuracy
- oil pipeline
- Hyperparameter tuning
- random forest
- Feature engineering
- Machine learning
Fuente:
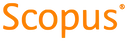
Tipo de documento:
Conference Object
Estado:
Acceso restringido
Áreas de conocimiento:
- Aprendizaje automático
- Ingeniería ambiental
Áreas temáticas de Dewey:
- Física aplicada
- Métodos informáticos especiales
- Ciencias de la computación

Objetivos de Desarrollo Sostenible:
- ODS 9: Industria, innovación e infraestructura
- ODS 12: Producción y consumo responsables
- ODS 3: Salud y bienestar
