Pbkp_rediction of Parkinson’s Disease Severity Based on Gait Signals Using a Neural Network and the Fast Fourier Transform
Abstract:
Parkinson’s disease (PD) is a chronic and progressive movement disorder affecting the nervous system of patients. PD alters the capacity to sustain a steady rhythm in the gait leading to impaired motor skills. The diagnosis of PD is a complex task that encompasses motor and non-motor symptoms. More than 50% of patients suffer from freezing in their legs during walking, generally defined as Freezing of Gait (FOG). Hence, gait evaluation is essential for physicians to detect the disease and track its severity. In this context, intelligent learning algorithms have been recently used to facilitate the diagnostic process. However, an accurate diagnosis is still required. This paper presents an effective and novel approach to diagnose PD using gait analysis, wearable sensors, and deep learning techniques. To achieve this goal, an Artificial Neural Network multiclassifier system (ANN) has been developed. The proposed model pbkp_redicts the severity of PD by detecting the stage of the disease. It uses a further analysis of movement alteration, supervised learning, and clinical assessment based on Hoehn & Yahr (H&Y) scores. The experimental results indicate a high efficiency of the proposed method in tracking PD severity. The method can make a distinction between idiopathic PD patients and healthy individuals, and classify them in the levels 0, 2, 2.5 and 3 according to the symptoms progress and level of disability. The model achieves an outstanding performance with an accuracy of 97%. Thus, this study emphasizes the use of a pbkp_rediction learning algorithm that can be helpful and valuable for clinical examinations.
Año de publicación:
2021
Keywords:
- Artificial Neural Network
- Fast fourier transform
- Gait Analysis
- Multiclassifier system
- Parkinson’s Disease
Fuente:
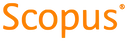
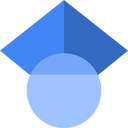
Tipo de documento:
Conference Object
Estado:
Acceso restringido
Áreas de conocimiento:
- Neurología
- Red neuronal artificial
Áreas temáticas:
- Enfermedades
- Fisiología humana
- Ciencias de la computación