Preprocessing of brain STEM auditory evoked potentials for diagnosing multiple sclerosis
Abstract:
Artificial neural networks, with radial basis functions (ANNRB), are used to diagnose patients with multiple sclerosis on the basis of their auditory evoked potentials. The wavelet transform coefficients of the evoked potentials are employed as inputs to the network. The paucity of the number of clinical cases available put a lot of restrictions on the network architecture. Only a handful of coefficients can be used to train the network, and we must select the coefficients that contain the most significant features [1].This feature selection is done using different statistical criteria, and we reduce the number to eight significant coefficients out of the hundreds or thousands of wavelet coefficients obtained in the transformation of the signals [2]. In a radial basis functions neural network, the number of free parameters jumps proportionally to the dimension of the input space when a new input node is added. A good selection of some of the centers for the radial basis functions will allow us to use more functions in the network architecture, making it more robust. To this end, we are going to use the power of unsupervised learning, before we apply the supervised one. In particular, we want to create clusters on the join input-output vector space of the supplied data utilizing a deterministic clustering algorithm. The centers and radii of these clusters could be employed for the centers and radii of the radial basis functions. To extract the maximum information from our preprocessing, we apply the locally most entropic estimate of the true join input-output probability density function to each of the obtained cluster-partitions and find the partition, and therefore the number of clusters, that has the lowest one [3]. The information from this partition, with its groups, centers and radii will be used to build the artificial network for diagnosing multiple sclerosis.
Año de publicación:
2006
Keywords:
- Multiple Sclerosis
- Neural networks
- wavelets
- Health Sciences
- Clustering algorithms
- Signal processing
Fuente:
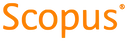
Tipo de documento:
Conference Object
Estado:
Acceso restringido
Áreas de conocimiento:
- Neurología
Áreas temáticas:
- Enfermedades
- Fisiología humana