Preserving empirical data utility in k-anonymous microaggregation via linear discriminant analysis
Abstract:
Today's countless benefits of exploiting data come with a hefty price in terms of privacy. k-Anonymous microaggregation is a powerful technique devoted to revealing useful demographic information of microgroups of people, whilst protecting the privacy of individuals therein. Evidently, the inherent distortion of data results in the degradation of its utility. This work proposes and analyzes an anonymization method that draws upon the technique of linear discriminant analysis (LDA), with the aim of preserving the empirical utility of data. Further, this utility is measured as the accuracy of a machine learning model trained on the microaggregated data. By transforming the original data records to a different data space, LDA enables k-anonymous microaggregation to build microcells more tailored to an intrinsic classification threshold. To do this, first, data is rotated (projected) towards the direction of maximum discrimination and, second, scaled in this direction by a factor α that penalizes distortion across the classification threshold. The upshot is that thinner cells are built along the threshold, which ends up preserving data utility in terms of the accuracy of machine learned models for a number of standardized data sets.
Año de publicación:
2020
Keywords:
- Statistical disclosure control
- Microaggregation
- Data utility
- Lda
- Data privacy
Fuente:
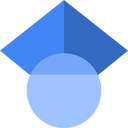
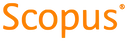
Tipo de documento:
Article
Estado:
Acceso restringido
Áreas de conocimiento:
- Minería de datos
- Optimización matemática
Áreas temáticas de Dewey:
- Programación informática, programas, datos, seguridad
- Biblioteconomía y Documentación informatica

Objetivos de Desarrollo Sostenible:
- ODS 9: Industria, innovación e infraestructura
- ODS 16: Paz, justicia e instituciones sólidas
- ODS 8: Trabajo decente y crecimiento económico
