A Semi-Supervised Approach for Microseisms Classification from Cotopaxi Volcano
Abstract:
Microseism classification is primordial to easily identify what type of event we are facing at in a possibly dangerous situation. However, labeling events is a hard and time-consuming task since it requires expert volcanologists to do this work. To alleviate the need for abundant labeled data, we propose a semi-supervised approach using the self-training algorithm. First, we extract several relevant microseisms features from the registers on the provided database, then we apply PCA to reduce redundancy on the features and finally we classify them using an SVM classifier. As a result of this methodology we show that although the accuracy of using a supervised scheme is still better than a semi-supervised one, if we allow a 10% of false positive rate, our approach achieves similar performance to supervised techniques with only 50% of labeled data. This demonstrates the potential of semi-supervised schemes.
Año de publicación:
2019
Keywords:
Fuente:
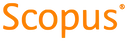
Tipo de documento:
Conference Object
Estado:
Acceso restringido
Áreas de conocimiento:
- Sismología
- Sismología
- Aprendizaje automático
Áreas temáticas:
- Geología, hidrología, meteorología
- Ciencias de la tierra