Probabilistic forecasting of drought events using Markov chain- and Bayesian network-based models: A case study of an Andean regulated river basin
Abstract:
The scarcity of water resources in mountain areas can distort normal water application patterns with among other effects, a negative impact on water supply and river ecosystems. Knowing the probability of droughts might help to optimize a priori the planning and management of the water resources in general and of the Andean watersheds in particular. This study compares Markov chain- (MC) and Bayesian network- (BN) based models in drought forecasting using a recently developed drought index with respect to their capability to characterize different drought severity states. The copula functions were used to solve the BNs and the ranked probability skill score (RPSS) to evaluate the performance of the models. Monthly rainfall and streamflow data of the Chulco River basin, located in Southern Ecuador, were used to assess the performance of both approaches. Global evaluation results revealed that the MC-based models predict better wet and dry periods, and BN-based models generate slightly more accurately forecasts of the most severe droughts. However, evaluation of monthly results reveals that, for each month of the hydrological year, either the MC- or BN-based model provides better forecasts. The presented approach could be of assistance to water managers to ensure that timely decision-making on drought response is undertaken.
Año de publicación:
2016
Keywords:
- Andean watersheds
- Markov chains
- Copulas
- Probabilistic drought forecasting
- Drought index
- Bayesian networks
Fuente:
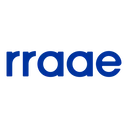
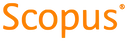
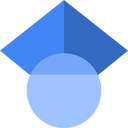
Tipo de documento:
Article
Estado:
Acceso abierto
Áreas de conocimiento:
- Hidrología
- Modelo estadístico
- Ciencia ambiental
Áreas temáticas de Dewey:
- Probabilidades y matemática aplicada
- Tecnología (Ciencias aplicadas)
- Otros problemas y servicios sociales

Objetivos de Desarrollo Sostenible:
- ODS 6: Agua limpia y saneamiento
- ODS 13: Acción por el clima
- ODS 15: Vida de ecosistemas terrestres
