Probabilistic solar forecasting benchmarks on a standardized dataset at Folsom, California
Abstract:
The present paper echos a recent data article, “A comprehensive dataset for the accelerated development and benchmarking of solar forecasting methods” [J. Renewable Sustainable Energy 11, 036102 (2019)]. The carefully composed dataset by Pedro, Larson, and Coimbra (PLC) presents a rare opportunity for solar forecasters to develop transparent and reproducible algorithms that can bring incremental contributions to the field. In their original paper, data from four different sources, namely, ground-based measurements, sky-camera images, satellite-imagery features, and numerical weather pbkp_rediction outputs, were arranged in a machine-learning-ready setup. Subsequently, several benchmarks for deterministic forecasting were set forth, for intra-hour, intra-day, and day-ahead scenarios. Nonetheless, a weather forecast is intrinsically five-dimensional, spanning space, time, and probability. In this regard, five reference methods for probabilistic forecasting: (1) complete-history persistence ensemble, (2) Markov-chain mixture model, (3) ordinary least squares, (4) analog ensemble, and (5) quantile regression, are applied to the PLC dataset. The R code provided in this paper follows the structure of the original Python code precisely, facilitating those solar forecasters who are not familiar with Python but have a statistics background.
Año de publicación:
2020
Keywords:
- NWP
- Probabilistic solar forecasting
- Satellite
- Standardized dataset
- Sky camera
Fuente:
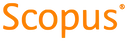
Tipo de documento:
Review
Estado:
Acceso restringido
Áreas de conocimiento:
- Análisis de datos
- Energía solar
- Estadísticas
Áreas temáticas:
- Física aplicada
- Educación, investigación, temas relacionados
- Otras ramas de la ingeniería