Proposing empirical correlations and optimization of Nu and S<inf>gen</inf> of nanofluids in channels and predicting them using artificial neural network
Abstract:
Getting the best performance from a thermal system requires two fundamental analyses, energy and entropy generation. An ideal mechanism has the highest Nu and the lowest entropy Sgen. As part of this research, a large dataset of fluid flow via tubes has been collected experimentally. As well as the inclusion of nanoparticles, analyses are included as well. By using deep learning algorithms, the Nusselt number and total entropy generation are predicted. In both models, the mean absolute error was lower than 5%. To determine the most accurate model, hyperparameter tuning is performed. That is adjusting all the settings in the neural network to attain the best results. The results of the predictive models are compared against experimental and benchmark results. The study incorporates a massive optimization strategy to fine-tune the predictive capabilities of the models. Furthermore, the model's predictive abilities are evaluated through the use of the coefficient of determination R2. For water and nanofluids flowing through circular, square, and rectangular cross-sections, the proposed models can predict Nu and Sgen. The results showed remarkable agreement with the experimental results. The models showed an MAE of not higher than 1.33%, which is a great achievement. Also, empirical correlations are proposed for both parameters, and double factorial optimization is implemented. The results showed that to achieve the best results, the Re should be higher than 1600, and the nanoparticle concentration should be 3%. A thorough justification of selected cases is presented as well.
Año de publicación:
2023
Keywords:
- Nusselt number
- artificial neural networks
- Entropy generation
- Machine learning
- Heat Transfer
- nanofluid
Fuente:
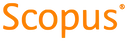
Tipo de documento:
Article
Estado:
Acceso abierto
Áreas de conocimiento:
- Optimización matemática
- Aprendizaje automático
- Matemáticas aplicadas
Áreas temáticas de Dewey:
- Física aplicada
- Métodos informáticos especiales
- Ciencias de la computación