A Systematic Literature Review of Learning-Based Traffic Accident Pbkp_rediction Models Based on Heterogeneous Sources
Abstract:
Statistics affirm that almost half of deaths in traffic accidents were vulnerable road users, such as pedestrians, cyclists, and motorcyclists. Despite the efforts in technological infrastructure and traffic policies, the number of victims remains high and beyond expectation. Recent research establishes that determining the causes of traffic accidents is not an easy task because their occurrence depends on one or many factors. Traffic accidents can be caused by, for instance, mechanical problems, adverse weather conditions, mental and physical fatigue, negligence, potholes in the road, among others. At present, the use of learning-based pbkp_rediction models as mechanisms to reduce the number of traffic accidents is a reality. In that way, the success of pbkp_rediction models depends mainly on how data from different sources can be integrated and correlated. This study aims to report models, algorithms, data sources, attributes, data collection services, driving simulators, evaluation metrics, percentages of data for training/validation/testing, and others. We found that the performance of a pbkp_rediction model depends mainly on the quality of its data and a proper data split configuration. The use of real data predominates over data generated by simulators. This work made it possible to determine that future research must point to developing traffic accident pbkp_rediction models that use deep learning. It must also focus on exploring and using data sources, such as driver data and light conditions, and solve issues related to this type of solution, such as high dimensionality in data and information imbalance.
Año de publicación:
2022
Keywords:
- deep learning
- neural network
- heterogeneous sources
- traffic accident pbkp_rediction
- Machine learning
Fuente:
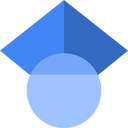
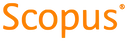
Tipo de documento:
Review
Estado:
Acceso abierto
Áreas de conocimiento:
- Aprendizaje automático
- Transporte
Áreas temáticas de Dewey:
- Otras ramas de la ingeniería
- Ciencias de la computación
- Otros problemas y servicios sociales