A View Invariant Human Action Recognition System for Noisy Inputs
Abstract:
We propose a skeleton-based Human Action Recognition (HAR) system, robust to both noisy inputs and perspective variation. This system receives RGB videos as input and consists of three modules: (M1) 2D Key-Points Estimation module, (M2) Robustness module, and (M3) Action Classification module; of which M2 is our main contribution. This module uses pre-trained 3D pose estimator and pose refinement networks to handle noisy information including missing points, and uses rotations of the 3D poses to add robustness to camera view-point variation. To evaluate our approach, we carried out comparison experiments between models trained with M2 and without it. These experiments were conducted on the UESTC view-varying dataset, on the i3DPost multi-view human action dataset and on a Boxing Actions dataset, created by us. Our system achieved positive results, improving the accuracy by 24%, 3% and 11% on each dataset, respectively. On the UESTC dataset, our method achieves the new state of the art for the cross-view evaluation protocols.
Año de publicación:
2022
Keywords:
- Robustness to noise
- Human pose
- View invariant
- Human Action Recognition
Fuente:
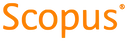
Tipo de documento:
Conference Object
Estado:
Acceso restringido
Áreas de conocimiento:
- Visión por computadora
- Ciencias de la computación
Áreas temáticas:
- Métodos informáticos especiales
- Ciencias de la computación
- Programación informática, programas, datos, seguridad