Providing entertainment by content-based filtering and reasoning in intelligent recommender systems
Abstract:
Recommender systems arose in view of the information overload present in numerous domains. The so-called content-based recommenders offer products similar to those the users liked in the past. However, due to the use of syntactic similarity metrics, these systems elaborate overspecialized recommendations including products very similar to those the user already knows. In this paper, we present a strategy that overcomes overspecialization by applying semantic reasoning techniques. Thanks to the reasoning, our strategy discovers huge amounts of knowledge about the user's preferences, and compares them with available products in a more flexible way, beyond the conventional syntactic metrics. The resulting reasoning-based strategy has been experimentally evaluated in the Digital TV domain. Our results show: (i) enhanced personalized recommendations, (ii) computational viability, and (iii) much greater accuracy. ©2008 IEEE.
Año de publicación:
2008
Keywords:
Fuente:
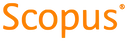
Tipo de documento:
Conference Object
Estado:
Acceso restringido
Áreas de conocimiento:
- Aprendizaje automático
- Ciencias de la computación
- Ciencias de la computación
Áreas temáticas de Dewey:
- Funcionamiento de bibliotecas y archivos
- Métodos informáticos especiales
- Ciencias de la computación

Objetivos de Desarrollo Sostenible:
- ODS 9: Industria, innovación e infraestructura
- ODS 17: Alianzas para lograr los objetivos
- ODS 8: Trabajo decente y crecimiento económico
