Q-Learning in a Multidimensional Maze Environment
Abstract:
Experiments with rodents in mazes demonstrate that, in addition to visual cues, spatial localization and olfactory sense play a key role in orientation, foraging and eventually survival. Simulation at some level and understanding of this unique behavior is important for solving optimal routing problems. This article proposes a Reinforcement Learning (RL) agent that learns optimal policies for discovering food sources in a 2D maze using space location and olfactory sensors. The proposed Q-learning solution uses a dispersion formula to generate a cheese smell matrix S, tied in space time to the reward matrix R and the learning matrix Q. RL is performed in a multidimensional maze environment, in which location and odor sensors cooperate in making decisions and learning optimal policies for foraging activities. The proposed method is computationally evaluated using location and odor sensor in two different scenarios: random and Deep-Search First (DFS), showing positive results in both cases.
Año de publicación:
2022
Keywords:
- Q-Learning
- Agent
- Multi-dimensional environment
- Maze solving
Fuente:
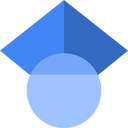
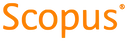
Tipo de documento:
Conference Object
Estado:
Acceso restringido
Áreas de conocimiento:
- Inteligencia artificial
- Algoritmo
- Ciencias de la computación
Áreas temáticas:
- Ciencias de la computación