QRS detection optimization in stress test recordings using evolutionary algorithms
Abstract:
QRS detection in exercise stress test recordings remains a challenging task, because they are highly non-stationary and contaminated with noises, such as large baseline wander and muscular noise, among others. The aim of this work is to find an optimal set of parameters for QRS detection in very noisy ECG signals, such as those acquired during stress tests. Parameter optimization was addressed by an evolutionary algorithm. A training database was created using 48 ECG recordings with reference QRS complexes. Each ECG recording is artificially contaminated with 3 types of real noise. A cost function combining the detection error probability, the mean detection jitter, and its standard deviation was defined, in order to obtain a quantitative performance evaluation of the detector. Evaluation was performed on an exercise stress test database composed of 54 real ECG recordings, with annotated QRS. The detector was configured with default parameter values, and also with the optimal values obtained from the evolutionary algorithm. The QRS detector with its optimized parameters showed a mean improvement of 4.6% compared to its performance with the default parameters. Furthermore, the use of optimized parameters led to at least the same performance than the initial parameters for all records, and the improvement was higher (up to 19.36 %) in noisy records, demonstrating the advantages of the optimized parameters in noisy environments.
Año de publicación:
2014
Keywords:
Fuente:
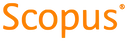
Tipo de documento:
Conference Object
Estado:
Acceso restringido
Áreas de conocimiento:
- Algoritmo
- Algoritmo
Áreas temáticas de Dewey:
- Fisiología humana

Objetivos de Desarrollo Sostenible:
- ODS 3: Salud y bienestar
- ODS 17: Alianzas para lograr los objetivos
- ODS 9: Industria, innovación e infraestructura
