Quantile regression for nonlinear mixed effects models: a likelihood based perspective
Abstract:
Longitudinal data are frequently analyzed using normal mixed effects models. Moreover, the traditional estimation methods are based on mean regression, which leads to non-robust parameter estimation under non-normal error distribution. However, at least in principle, quantile regression (QR) is more robust in the presence of outliers/influential observations and misspecification of the error distributions when compared to the conventional mean regression approach. In this context, this paper develops a likelihood-based approach for estimating QR models with correlated continuous longitudinal data using the asymmetric Laplace distribution. Our approach relies on the stochastic approximation of the EM algorithm (SAEM algorithm), obtaining maximum likelihood estimates of the fixed effects and variance components in the case of nonlinear mixed effects (NLME) models. We evaluate the finite sample performance of the SAEM algorithm and asymptotic properties of the ML estimates through simulation experiments. Moreover, two real life datasets are used to illustrate our proposed method using the qrNLMM package from R.
Año de publicación:
2020
Keywords:
- Nonlinear mixed effects models
- Quantile regression
- Asymmetric Laplace distribution
- SAEM algorithm
Fuente:
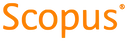
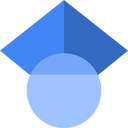
Tipo de documento:
Article
Estado:
Acceso restringido
Áreas de conocimiento:
- Estadísticas
- Optimización matemática
Áreas temáticas:
- Programación informática, programas, datos, seguridad
- Probabilidades y matemática aplicada
- Fisiología humana