Quantitative structure–activity relationship analysis and virtual screening studies for identifying HDAC2 inhibitors from known HDAC bioactive chemical libraries
Abstract:
Histone deacetylases (HDAC) are emerging as promising targets in cancer, neuronal diseases and immune disorders. Computational modelling approaches have been widely applied for the virtual screening and rational design of novel HDAC inhibitors. In this study, different machine learning (ML) techniques were applied for the development of models that accurately discriminate HDAC2 inhibitors form non-inhibitors. The obtained models showed encouraging results, with the global accuracy in the external set ranging from 0.83 to 0.90. Various aspects related to the comparison of modelling techniques, applicability domain and descriptor interpretations were discussed. Finally, consensus pbkp_redictions of these models were used for screening HDAC2 inhibitors from four chemical libraries whose bioactivities against HDAC1, HDAC3, HDAC6 and HDAC8 have been known. According to the results of virtual screening assays, structures of some hits with pair-isoform-selective activity (between HDAC2 and other HDACs) were revealed. This study illustrates the power of ML-based QSAR approaches for the screening and discovery of potent, isoform-selective HDACIs.
Año de publicación:
2017
Keywords:
- Machine learning
- quantitative structure–activity relationship
- chemoinformatics
- Histone deacetylase inhibitor
- Virtual Screening
Fuente:
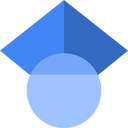
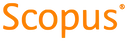
Tipo de documento:
Article
Estado:
Acceso restringido
Áreas de conocimiento:
- Descubrimiento de fármacos
- Bioquímica
- Farmacología
Áreas temáticas:
- Programación informática, programas, datos, seguridad
- Farmacología y terapéutica
- Bioquímica