Rainfall Pbkp_redictive Approach for la Trinidad, Benguet using Machine Learning Classification
Abstract:
Use of rain as a source of irrigation water presents an effective use of natural water resources. Pbkp_redicting the occurrence of rainfall plays a major role especially in an agricultural area with untimely rainfall like La Trinidad, Benguet. For a more efficient irrigation scheduling, a reliable method for rainfall pbkp_rediction is needed. This entails the adaptation and utilization of suitable pbkp_rediction approaches and techniques. Various analytical approaches and methods are made available to develop new techniques to pbkp_redict future possibilities. This study aimed to propose an approach in pbkp_redicting the occurrence and non-occurrence of rainfall in La Trinidad, Benguet based on various historical weather parameters. Five machine learning classification algorithms were used to build the pbkp_redictive models for the weather dataset namely: Fine Decision Tree, Linear Discriminant, Course K-Nearest Neighbors, Gaussian Support Vector Machines, and Neural Network. A poor choice of model cannot further improve the pbkp_redictions. To choose between models, focus must be put on the appropriate evaluation metrics. Among the 5 models, results suggest that Course K-Nearest Neighbor gives the highest performance in all the evaluation metrics. Course KNN, with a good accuracy of 81.1% proves to be the best model to use in pbkp_redicting rainfall in La Trinidad, Benguet. Course KNN model evaluation reveals that Machine Learning Classification can be adopted to pbkp_redict the occurrence and non-occurrence of rainfall.
Año de publicación:
2019
Keywords:
- performance metrics
- pbkp_redictive models
- Classifiers
- Machine learning
- rain pbkp_rediction
Fuente:
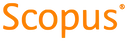
Tipo de documento:
Conference Object
Estado:
Acceso restringido
Áreas de conocimiento:
- Simulación por computadora
- Aprendizaje automático
- Ciencias de la computación
Áreas temáticas de Dewey:
- Ciencias de la computación