Rapid Detection of Cardiac Pathologies by Neural Networks Using ECG Signals (1D) and sECG Images (3D)
Abstract:
Usually, cardiac pathologies are detected using one-dimensional electrocardiogram signals or two-dimensional images. When working with electrocardiogram signals, they can be represented in the time and frequency domains (one-dimensional signals). However, this technique can present difficulties, such as the high cost of private health services or the time the public health system takes to refer the patient to a cardiologist. In addition, the variety of cardiac pathologies (more than 20 types) is a problem in diagnosing the disease. On the other hand, surface electrocardiography (sECG) is a little-explored technique for this diagnosis. sECGs are three-dimensional images (two dimensions in space and one in time). In this way, the signals were taken in one-dimensional format and analyzed using neural networks. Following the transformation of the one-dimensional signals to three-dimensional signals, they were analyzed in the same sense. For this research, two models based on LSTM and ResNet34 neural networks were developed, which showed high accuracy, 98.71% and 93.64%, respectively. This study aims to propose the basis for developing Decision Support Software (DSS) based on machine learning models.
Año de publicación:
2022
Keywords:
- matlab
- PYTHON
- LSTM
- sECG images
- ResNet34
- Neural networks
Fuente:
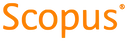
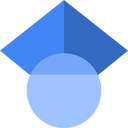
Tipo de documento:
Article
Estado:
Acceso abierto
Áreas de conocimiento:
- Enfermedad cardiovascular
- Red neuronal artificial
- Ciencias de la computación
Áreas temáticas:
- Enfermedades
- Medicina y salud
- Ciencias de la computación