Real-Time transient stability assessment of electric power systems using pbkp_redictive-sime based on machine learning
Abstract:
This paper presents a novel methodology based on machine learning for assessing the power system transient stability (TS) in real-Time using synchronized phasor measurements as input in order to pbkp_redict the power system TS status related to the single machine equivalent (SIME) results. Based on the probabilistic models of input parameters, such as load variation and the occurrence of contingencies, Monte Carlo-Type simulation is performed off-line to iteratively evaluate the system TS responses using the SIME methodology. Afterwards, the database obtained from the off-line simulations is employed for structuring and training an intelligent SIME's TS margin classifier based on support vector machines (SVM) to be used for real-Time transient stability assessment (TSA). Besides, the SVM is optimally tuned by using the swarm variant of the mean-variance mapping optimization MVMOS. Several tests are then performed on the New England benchmark power system. Results demonstrate the feasibility and effectiveness that could be achieved in estimating the transient stability status related to the SIME's TS margins, which will also be of great value for defining suitable emergency control actions in real time.
Año de publicación:
2017
Keywords:
- SIME
- Support Vector Machine
- Transient Stability.
- Machine learning
- Monte Carlo
Fuente:
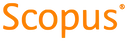
Tipo de documento:
Conference Object
Estado:
Acceso restringido
Áreas de conocimiento:
- Potencia eléctrica
- Aprendizaje automático
Áreas temáticas:
- Física aplicada
- Ciencias de la computación
- Métodos informáticos especiales