Real-time Social Distancing Detection Approach Using YOLO and Unmanned Aerial Vehicles
Abstract:
The current COVID-19 pandemic has undoubtedly brought new challenges to society and the constant search for solutions to control and reduce its effects. In this sense, the use of technology has become essential to cope with the situation. Thus, this work proposes a real-time social distancing detection system using Deep Learning algorithms and carrying out the monitoring through a UAV. This system consists of two fundamental blocks. The first one consists of convolutional neural network training to detect people using the YOLO object detection system while the second one consists of real-time video acquisition and analysis. Practical applications involves detecting people and calculating the distances between them to determine whether social distancing measurements are being obeyed or not. By increasing surveillance capabilities, authorities and security forces may control and prevent possible outbreaks of massive COVID-19 infections. The experiments were made in three different flight scenarios with altitudes of 15, 30, and 50 m. According to the results, the detection system’s recall reaches values close to 90%, although the highest values were obtained in flights at 30 m high. Regarding the calculation of the distances, in the three scenarios, the average relative error did not exceed 5%. Thus, the video transmission showed a high performance during the experiments. Hence, the system returns reliable results to control compliance with measures such as social distancing.
Año de publicación:
2022
Keywords:
- covid-19
- cnn
- deep learning
- YOLO
- Computer Vision
- UAV
Fuente:
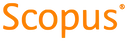
Tipo de documento:
Conference Object
Estado:
Acceso restringido
Áreas de conocimiento:
- Visión por computadora
- Ciencias de la computación
Áreas temáticas:
- Métodos informáticos especiales