Recognition of human hand activities based on a single wrist imu using recurrent neural networks
Abstract:
Recognition of hand activities could provide new information towards daily human activity logging and gesture interface applications. However, there is a technical challenge due to delicate hand motions and complex movement contexts. In this work, we proposed hand activity recognition (HAR) based on a single inertial measurement unit (IMU) sensor at one wrist via deep learning recurrent neural network. The proposed HAR works directly with signals from a tri-axial accelerometer, gyroscope, and magnetometer sensors within one IMU. We evaluated the performance of our HAR with a public human hand activity database for six hand activities including Open Door, Close Door, Open Fridge, Close Fridge, Clean Table and Drink from Cup. Our results show an overall recognition accuracy of 80.09% with discrete standard epochs and 74.92% with noise-added epochs. With continuous time series epochs, the accuracy of 71.75% was obtained.
Año de publicación:
2017
Keywords:
Fuente:
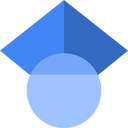
Tipo de documento:
Other
Estado:
Acceso abierto
Áreas de conocimiento:
- Aprendizaje automático
- Ciencias de la computación
Áreas temáticas:
- Métodos informáticos especiales
- Ciencias de la computación
- Ingeniería y operaciones afines