Reconciling solar forecasts: Probabilistic forecasting with homoscedastic Gaussian errors on a geographical hierarchy
Abstract:
Hierarchical forecasting and reconciliation are new to the field of solar engineering. Previous papers in this series, namely, Yang et al. (2017a, 2017b), and Yagli et al. (2019b), discussed various reconciliation techniques for deterministic solar forecasts obtained across spatio-temporal hierarchies. This paper extends the discussion into probability space, and studies how reconciliation can affect the performance of probabilistic forecasting. More specifically, qualities of the parametric pbkp_redictive distributions before and after reconciliation are compared. Four minimum-trace-based reconciliation techniques are used to reconcile day-ahead and hour-ahead forecasts generated using two datasets: (1) distributed solar power generation for 318 simulated PV systems in California, and (2) satellite-derived irradiance over Arizona. The empirical result shows that reconciliation not only improves the accuracy of point forecasts, but also leads to high-quality pbkp_redictive distributions in terms of sharpness, calibration, and skill score. Moreover, such improvement is quite general, and does not seem to depend on data, hierarchy structure, nor the underlying forecasting model.
Año de publicación:
2020
Keywords:
- Reconciliation
- Machine learning
- Numerical weather pbkp_rediction
- Satellite-derived irradiance
- solar forecasting
- probabilistic forecasting
Fuente:
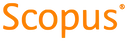
Tipo de documento:
Article
Estado:
Acceso restringido
Áreas de conocimiento:
- Energía renovable
- Estadísticas
- Big data
Áreas temáticas:
- Probabilidades y matemática aplicada
- Geología, hidrología, meteorología
- Física aplicada