Regression Based Machine Learning to Generate and Validate a Metric for Food Insecurity
Abstract:
With the increased cost of living, exacerbated by COVID-19, thousands in New Jersey lack secure access to nutritious food. Given the importance of the issue, this research aims to produce an accurate metric using accessible data to quantify food insecurity. 16 potential explanatory variables, such as median household income and homeless population, were chosen as their values were defined across all NJ counties from 2015-2019. Using multiple linear regression, 14 unique metrics were created after four different variable pruning methods. The leading metric, with an adj. R2 value of 0.932, demonstrates the correlation between food insecurity, population, median household income, total population with health insurance, and population with private health insurance. The implementation of this metric could serve as a tool in pbkp_redicting areas of food insecurity, highlighting affiliated factors, and revealing connections between racial populations.
Año de publicación:
2021
Keywords:
- food insecurity
- Machine learning
- pbkp_rediction model
- PYTHON
- New Jersey
- data science
Fuente:
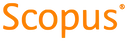
Tipo de documento:
Conference Object
Estado:
Acceso restringido
Áreas de conocimiento:
- Seguridad alimentaria
- Aprendizaje automático
Áreas temáticas:
- Ciencias de la computación