Reinforcement Learning for Estimating Student Proficiency in Math Word Problems
Abstract:
Math Word Problems (MWPs) allow preparing students to apply mathematical skills in everyday life. Teaching MWPs is challenging because the mathematics difficulties of individual students vary across students within a class. In this paper, it is proposed a reinforcement learning algorithm based on Q-learning for estimating individual student proficiency according to the basic arithmetic operations: addition, subtraction, multiplication, and division. The proposal is analyzed by simulating interactions with hand-coded users but tested by simulating interactions with data-driven users. In addition, results are compared with those in a common Cognitive Diagnosis Model (CDM). Results show that our approach allows estimating the individual student proficiency in around 5 episodes. Thus, since reinforcement learning usually is applied to induce instructional policies in tutoring systems, this paper shows that it can be used for estimating student proficiency as well.
Año de publicación:
2022
Keywords:
- reinforcement learning
- student proficiency
- math word problems
- mathematics difficulties
Fuente:
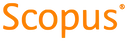
Tipo de documento:
Conference Object
Estado:
Acceso restringido
Áreas de conocimiento:
- Aprendizaje automático
- Tecnología educativa
Áreas temáticas:
- Matemáticas
- Educación
- Escuelas y sus actividades; educación especial