Reinforcement learning based energy management algorithm for energy trading and contingency reserve application in a microgrid
Abstract:
This paper presents automation of energy trading using a value-based reinforcement learning and Deep-Q-Network based energy management algorithm for microgrid participants having energy storage systems, while maintaining required contingency reserves for the microgrid. A piecewise utility function is designed to form the trading strategies in response to the dynamic environment. By adjusting parameters of the utility function, two different behaviors of the microgrid operator, namely risk-seeking and risk-averse, are studied to analyze the impact on the energy storage's operation decision. The simulation results show that the proposed algorithm manages the storage optimally and outperforms rule-based algorithm, providing higher monetary benefits and better flexibility during an extreme scenario having highly dynamic pricing.
Año de publicación:
2020
Keywords:
- Contingency reserve
- microgrid
- Dynamic pricing
- energy management
- Model-free reinforcement learning
Fuente:
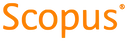
Tipo de documento:
Conference Object
Estado:
Acceso restringido
Áreas de conocimiento:
- Aprendizaje automático
- Energía
- Energía
Áreas temáticas:
- Ciencias de la computación
- Física aplicada