Reliable object recognition by using cooperative neural agents
Abstract:
An artificial vision system based upon known insect brain structures is presented. It reliably recognizes real world objects visualized through a web cam or read from databases, and utilizes neural agents that communicate through time stabilized sparse code. A three layer ANN is trained to track one reticle pattern. Once trained the net becomes a proactive agent by participating in a local, close loop control system which oscillates, shows a sturdy emergent tracking behavior and produces a continuous flow of space-time related unstable code. This flow is time stabilized, converted to sparse form and relayed to a population of other isolated neural agents, whose response can be tuned to complex visual stimulus. Finally a novel noise-balanced training method is used to tune agents' response in and secluded environment, where only the images of a chosen object and noise exist. Isolation creates a strong agent-object association that boosts object recognition. The found solutions sustain sparse code, visual invariance and concentrate their decision into a single neuron. These might represents good start up conditions for modeling concept cells. The system has been tested using real time real world images and data bases.
Año de publicación:
2014
Keywords:
- concept cell
- Object recognition
- Computer Vision
- isolated learning
- cooperative agents
Fuente:
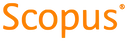
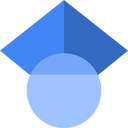
Tipo de documento:
Conference Object
Estado:
Acceso restringido
Áreas de conocimiento:
- Red neuronal artificial
- Ciencias de la computación
- Ciencias de la computación
Áreas temáticas:
- Métodos informáticos especiales