ResU-Net: Residual convolutional neural network for prostate MRI segmentation
Abstract:
The identification and segmentation of the prostate on magnetic resonance images (MRI) can assist in the diagnosis of prostate diseases, and improve image-guided intervention. However, prostate segmentation is normally performed manually resulting in a time-consuming process that delays the treatment. Therefore, automating the prostate segmentation process is needed to improve the pbkp_rediction and treatment of prostate diseases. Segmenting the prostate on MRI is challenging due to the lack of clear boundaries between the prostate and neighboring tissues, the variability among images acquired through different protocols, and the inherent variability of the shape and size of the prostate among patients. In this paper, we present a new deep convolutional neural network architecture called ResU-Net that can automatically identify and segment the prostate on MRI. The proposed ResU-Net architecture has a similar structure to the well-known U-net but uses the residual learning framework as the building block to increase the dissemination of information to deeper layers and to overcome the challenging vanishing gradient problem. The model is tested in a publically available dataset and produces a high segmentation accuracy. Additionally, the model's use of residual connections and data augmentation enables it to generalize well even with a restricted amount of annotated images.
Año de publicación:
2018
Keywords:
- deep learning
- medical image processing
- image segmentation
- convolutional neural networks
Fuente:
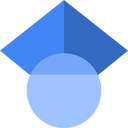
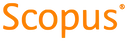
Tipo de documento:
Conference Object
Estado:
Acceso restringido
Áreas de conocimiento:
- Aprendizaje automático
- Ciencias de la computación
Áreas temáticas:
- Ciencias de la computación