Reservoir computing meets smart grids: Attack detection using delayed feedback networks
Abstract:
A new method for attack detection of smart grids with wind power generators using reservoir computing (RC) is introduced in this paper. RC is an energy-efficient computing paradigm within the field of neuromorphic computing and the delayed feedback networks (DFNs) implementation of RC has shown superior performance in many classification tasks. The combination of temporal encoding, DFN, and a multilayer perceptron (MLP) as the output readout layer is shown to yield performance improvement over existing attack detection methods such as MLPs, support vector machines (SVM), and conventional state vector estimation (SVE) in terms of attack detection in smart grids. The proposed algorithms are shown to be more robust than MLP and SVE in dealing with different variables such as the amplitude of the attack, attack types, and the number of compromised measurements in smart grids. The attack detection rate for the proposed RC-based system is higher than 99%, based on the accuracy metric for the average of 10 000 simulations.
Año de publicación:
2018
Keywords:
- Reservoir computing (RC)
- Neuromorphic computing
- Temporal encoder
- smart grids
- Delayed feedback networks (DFNs)
- Attack detection
- State vector estimation (SVE)
Fuente:
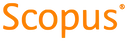
Tipo de documento:
Article
Estado:
Acceso abierto
Áreas de conocimiento:
- Aprendizaje automático
- Ciencias de la computación
- Simulación por computadora
Áreas temáticas:
- Física aplicada