A closed-loop data-driven optimization framework for the unit commitment problem: A Q-learning approach under real-time operation
Abstract:
Real-time operation of electric power systems under high penetration of renewable energy generation has to incorporate strategies for managing the uncertainty associated with this type of power sources. In the case of the unit commitment problem, models based on robust optimization have been widely used for the day-ahead computation of power dispatch and reserves schedule. Typical approaches use uncertainty sets with fixed levels of conservativeness, without considering the real-time performance of the solutions. This paper proposes a solution scheme for the adaptive robust unit commitment problem using online adjusted data-driven uncertainty sets. Conservativeness parameters of the uncertainty sets are dynamically calculated as a function of previous operating results and incoming data via reinforcement learning, resulting in an online learning-optimization framework. The paper also develops an experimental framework to simulate real-time operation under the proposed methodology. Out-of-sample experiments illustrate the effectiveness of the proposed scheme against well-known benchmarks with fixed robustness levels. Results show improvements in power generation costs, voltage violations, and use of reserves. Two systems of different sizes are analyzed, illustrating the scalability of the proposed approach.
Año de publicación:
2023
Keywords:
- unit commitment
- Robust optimization
- Online optimization
- reinforcement learning
- Data-driven uncertainty set
Fuente:
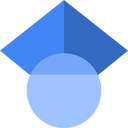
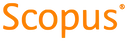
Tipo de documento:
Article
Estado:
Acceso restringido
Áreas de conocimiento:
- Inteligencia artificial
- Optimización matemática
- Optimización matemática
Áreas temáticas:
- Sistemas