Reward Shaping to Learn Natural Object Manipulation With an Anthropomorphic Robotic Hand and Hand Pose Priors via On-Policy Reinforcement Learning
Abstract:
A key challenge in reinforcement learning (RL) for robot manipulation is to provide a reward function that allows reliable and stable learning to achieve their goals while interacting with the environment. Unfortunately, rewards are usually task-specific, and their engineering is challenging and laborious especially for an anthropomorphic robotic hand with high degrees of freedom. In this work, we consider a reward function for learning a policy under the constrain of minimizing the robot hand pose to demonstration priors. We propose a shaped reward for obtaining efficient manipulation policies after incorporating five-fingered hand poses of grasping demonstrations for various objects into the early timesteps of the training episodes. The trained policy NPG+SR with our proposed reward improves the average success rate over 95% for grasping and relocating all objects compared to 68% obtained with the baseline NPG-B. We noticed that our method not only performs better but the qualitative results indicate that for the objects such as an apple, water bottle, and lightbulb incorporating hand pose priors for learning allows a more natural hand grasping.
Año de publicación:
2021
Keywords:
- Anthropomorphic robotic hand
- Hand Poses Priors
- Deep reinforcement learning
- object manipulation
Fuente:
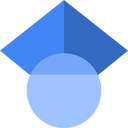
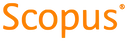
Tipo de documento:
Conference Object
Estado:
Acceso restringido
Áreas de conocimiento:
- Robótica
Áreas temáticas:
- Ciencias de la computación