Roles of CCG crossover and mutation in evolutionary many-objective optimization
Abstract:
When we solve many-objective optimization problems (MaOPs) by using multi-objective evolutionary algorithms (MOEAs), genetic diversity of solutions in the population significantly increases in order to explore the true Pareto optimal solutions widely distributed in variable space. In MOEAs, if solutions in the population become noticeably diverse in variable space, conventional crossovers become too disruptive genetic operator and decrease its effectiveness. To overcome this problem in MaOPs, crossover controlling the number of crossed genes (CCG) has been proposed. CCG controls the number of crossed genes by using an user-defined parameter α. CCG with small α significantly improves the search performance of MOEAs in MaOPs by keeping small the number of crossed genes. CCG operator has a similar feature to mutation operator in the sense that both operators can control the amount of genetic variation to create offspring. To clarify the roles of CCG crossover and mutation in MaOPs, in this work we analyze the search performance of CCG-only, mutation-only and combined CCG-mutation based search on many-objective 0/1 knapsack problems. Simulation results reveal that mutation induces diversity in the Pareto front and CCG crossover enhances convergence towards the true Pareto front. Also, we show that highest search performance, realizing well-balanced search between convergence and diversity, can be achieved by combined CCG-mutation rather than by mutation-only or CCG-only based search. © 2012 TSI Press.
Año de publicación:
2012
Keywords:
Fuente:
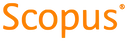
Tipo de documento:
Conference Object
Estado:
Acceso restringido
Áreas de conocimiento:
- Evolución
- Algoritmo
Áreas temáticas:
- Programación informática, programas, datos, seguridad
- Miscelánea filosófica
- Economía financiera
- Dirección general