Rotary dryer control using a Grey-Box Neural Model scheme
Abstract:
The application of a Grey-box Neural Model (GNM) in a nonlinear model pbkp_redictive control scheme (NMPC) of a direct rotary dyer is presented in this work. The GNM, which is based on the combination of phenomenological models and empirical artificial neural network (ANN) models, was properly developed and validated by using experimental fish-meal rotary drying information. The GNM was created by combining the rotary dryer mass and energy balances and a feed forward neural network (FFNN), trained off-line to estimate the drying rate and the volumetric heat transfer coefficient. The GNM results allowed us to obtain the relation between the controlled variable (solid moisture content) and the manipulated variable (gas phase entrance temperature) used in the pbkp_redictive control strategy. Two NMPC control strategies, one with a fixed extended pbkp_rediction horizon and another with an extended range pbkp_rediction horizon, were applied to a simulated industrial fish-meal drying process. The results showed that a correct rotary dryer representation can be obtained by using a GNM approach. Due to the representation capability of the GNM approach, excellent control performances of the NMPCs were observed when the process variables were subject to disturbances. As analyzed in this work, the fixed extended pbkp_rediction horizon MPC surpassed recognized control methodologies (quadratic dynamic matrix control). © 2011 Taylor & Francis Group, LLC.
Año de publicación:
2011
Keywords:
- Dryer control
- Nonlinear model pbkp_redictive control
- Drying modeling
Fuente:
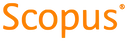
Tipo de documento:
Article
Estado:
Acceso restringido
Áreas de conocimiento:
- Simulación por computadora
- Red neuronal artificial
Áreas temáticas:
- Física aplicada
- Métodos informáticos especiales
- Ciencias de la computación