SVM and SSD for Classification of Mobility Actors on the Edge
Abstract:
In the context of video processing, transmission to a remote server is not always possible nor suitable. Video processing on the edge could offer a solution. However, lower processing capacities constraint the number of techniques available for devices, in this work we report the performance of two techniques for classification from video on a minicomputer. The implementation of a real-time vehicle counting and classification system is evaluated through Support Vector Machine (SVM) and the Single Shot Detector Framework (SSD) in a minicomputer. We compare two SVM bases techniques, IPHOG and a MBF using Scale Invariant Features. The obtained results show that with a video resolution of 1280 × 720 pixels and using SVM, precision and recognition rates of 86% and 94% are obtained respectively, while with SSD 93% and 67% rates are reached with times of processing higher than SVM.
Año de publicación:
2019
Keywords:
- Raspberry PI
- Convolutional neural network
- Performance evaluation
- Support Vector Machine
- Computer Vision
- Single Shot Detection
Fuente:
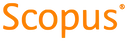
Tipo de documento:
Conference Object
Estado:
Acceso restringido
Áreas de conocimiento:
- Aprendizaje automático
- Ciencias de la computación
Áreas temáticas de Dewey:
- Métodos informáticos especiales

Objetivos de Desarrollo Sostenible:
- ODS 9: Industria, innovación e infraestructura
- ODS 11: Ciudades y comunidades sostenibles
- ODS 8: Trabajo decente y crecimiento económico
