Sales Forecast by Using Deep Rectifier Network
Abstract:
Sales forecast is a key issue in pharmacy wholesales and has a direct effect in inventory cost, it also represents a difficult problem, affected by factors like promotions, price changes and seasonal preferences. Traditional sales forecast techniques use historical sales data and recursive formulas, which limit their accuracy. More recently learning-based methods have expanded data features capture capabilities. This paper presents a deep architecture which explores a few years of weekly sales data and learns to makes assertive pbkp_redictions. The system is assembled with ReLU neurons, whose learning behavior makes possible the effective training of sparse coded deep sub-nets. The developed learning algorithm uses two learning stages where the first produces sparse representation of the studied time series and the second harvest one week ahead pbkp_redictions using this sparse data. In both cases the reward system is future-focused and favors search for future capacities. To achieving successful network training we develop an algorithms that deals with exploding gradient problem, typical of ReLU networks. The fully assembled pbkp_redictor automatically learn features from structured data and produces inventories with improved dollar cost. The system has been tested in real time with real data.
Año de publicación:
2021
Keywords:
- ReLU Neurons
- Sale forecast
- deep learning
Fuente:
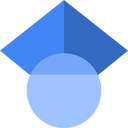
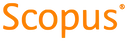
Tipo de documento:
Conference Object
Estado:
Acceso restringido
Áreas de conocimiento:
- Aprendizaje profundo
- Algoritmo
Áreas temáticas:
- Sistemas
- Métodos informáticos especiales
- Dirección general