Scada data-driven wind turbine main bearing fault prognosis based on one-class support vector machines
Abstract:
This work proposes a fault prognosis methodology to pbkp_redict the main bearing fault several months in advance and let turbine operators plan ahead. Reducing downtime is of paramount importance in wind energy industry to address its energy loss impact. The main advantages of the proposed methodology are the following ones. It is an unsupervised approach, thus it does not require faulty data to be trained; ii) it is based only on exogenous data and one representative temperature close to the subsystem to diagnose, thus avoiding data contamination; iii) it accomplishes the prognosis (various months in advance) of the main bearing fault; and iv) the validity and performance of the established methodology is demonstrated on a real underproduction wind turbine.
Año de publicación:
2021
Keywords:
- Wind turbine
- Fault prognosis
- SCADA data
- Main bearing
Fuente:
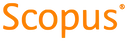
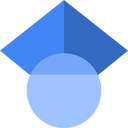
Tipo de documento:
Article
Estado:
Acceso abierto
Áreas de conocimiento:
- Software
- Aprendizaje automático
Áreas temáticas:
- Métodos informáticos especiales
- Física aplicada