Scalable Solution Strategies for Chance-Constrained Nonlinear Programs
Abstract:
Probabilistic (chance) constraints are a powerful modeling paradigm that helps decision-makers control risk. Unfortunately, chance constraints (CCs) cannot be handled directly by off-the-shelf optimization solvers and specialized reformulations and solution procedures are often needed. In this work, we review different strategies to tackle large-scale nonlinear programs (NLPs) with CCs. In particular, we use moment matching when the algebraic structure of the moments and of the quantile function of the CC distribution are known. To address more general settings with arbitrary distributions, we use a sigmoidal approximation, which provides a mechanism to achieve exact solutions. We demonstrate that this approach significantly reduces the conservatism of popular approximations such as the conditional value at risk and the scenario (almost surely) approach. A flare system design study is used to illustrate the developments.
Año de publicación:
2018
Keywords:
Fuente:
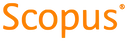
Tipo de documento:
Article
Estado:
Acceso restringido
Áreas de conocimiento:
- Optimización matemática
- Optimización matemática
- Optimización matemática
Áreas temáticas:
- Sistemas
- Probabilidades y matemática aplicada
- Gestión y servicios auxiliares