A comparative analysis of similarity metrics on sparse data for clustering in recommender systems
Abstract:
This work shows similarity metrics behavior on sparse data for recommender systems (RS). Clustering in RS is an important technique to perform groups of users or items with the purpose of personalization and optimization recommendations. The majority of clustering techniques try to minimize the Euclidean distance between the samples and their centroid, but this technique has a drawback on sparse data because it considers the lack of value as zero. We propose a comparative analysis of similarity metrics like Pearson Correlation, Jaccard, Mean Square Difference, Jaccard Mean Square Difference and Mean Jaccard Difference as an alternative method to Euclidean distance, our work shows results for FilmTrust and MovieLens 100K datasets, these both free and public with high sparsity. We probe that using similarity measures is better for accuracy in terms of Mean Absolute Error and Within-Cluster on sparse data.
Año de publicación:
2019
Keywords:
- Clustering
- recommender systems
- Similarity Measures
Fuente:
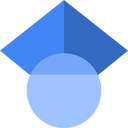
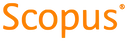
Tipo de documento:
Conference Object
Estado:
Acceso restringido
Áreas de conocimiento:
- Minería de datos
- Ciencias de la computación
- Ciencias de la computación
Áreas temáticas:
- Funcionamiento de bibliotecas y archivos