Seawater intrusion pattern recognition supported by unsupervised learning: A systematic review and application
Abstract:
Seawater intrusion is among the world's leading causes of groundwater contamination, as salty water can affect potable water access, food production, and ecosystem functions. To explore such contamination sources, multivariate analysis supported by unsupervised learning tools has been used for decades to aid in water resource pattern recognition, clustering, and water quality data variability characterization. This study proposes a systematic review of these techniques applied for supporting seawater intrusion identification based on the Preferred Reporting Items for Systematic Reviews and Meta-Analyses (PRISMA) statement and subsequent bibliometric analysis of 102 coastal hydrogeological studies. The most relevant identified methods, including principal components analysis (PCA), hierarchical clustering analysis, K-means clustering, and self-organizing maps, are explained and applied to a case study. Although 74 % of the studies that applied dimensional reduction methods, such as PCA, associated most of the database variance with the salinization process, 77 % of the studies that applied clustering methods associated at least one water sample cluster with the influence of seawater intrusion. Based on the review and a practical demonstration using the open-source R software platform, recommendations are made regarding data preprocessing, research opportunities, and publishing information necessary to replicate and validate the studies.
Año de publicación:
2023
Keywords:
- multivariate
- Clustering
- Coastal aquifers
- Machine learning
- Salinization
Fuente:
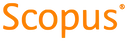
Tipo de documento:
Review
Estado:
Acceso abierto
Áreas de conocimiento:
- Hidrología
- Aprendizaje automático
Áreas temáticas de Dewey:
- Métodos informáticos especiales
- Biblioteconomía y Documentación informatica
- Ciencias Naturales y Matemáticas