Seismic Event Classification Using Spectrograms and Deep Neural Nets
Abstract:
In this work, we proposed a new method to classify long-period and volcano-tectonic spectrogram images using eight different deep learning architectures. The developed method used three deep convolutional neural networks named DCNN1, DCNN2, and DCNN3, three deep convolutional neural networks combined with deep recurrent neural networks named DCNN-RNN1, DCNN-RNN2, and DCNN-RNN3, and two autoencoder neural networks named AE1 and AE2, to maximize the area under the curve of the receiver operating characteristic scores on a dataset of volcano seismic spectrogram images. The three deep recurrent neural network-based models reached the worst results due to the overfitting produced by the small number of samples in the training sets. The DCNN1 overcame the remaining models by obtaining an area under the curve of the receiver operating characteristic and accuracy scores of 0.98 and 95 %, respectively. Although these values were not the highest values per metric, they did not represent statistical differences against other results obtained by more algorithmically complex models. The proposed DCNN1 model showed similar or superior performance compared to the majority of the state of the art methods in terms of accuracy. Therefore it can be considered a successful scheme to classify LP and VT seismic events based on their spectrogram images.
Año de publicación:
2021
Keywords:
- volcanic seismic event classification
- Artificial Intelligence
- spectrogram images
- deep-learning models
Fuente:
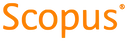
Tipo de documento:
Conference Object
Estado:
Acceso restringido
Áreas de conocimiento:
- Sismología
- Aprendizaje automático
- Sismología
Áreas temáticas:
- Geología, hidrología, meteorología
- Física aplicada
- Ciencias de la computación