Selecting and combining models with self-organizing maps for long-term forecasting of chaotic time series
Abstract:
When time series are generated by chaotic systems, a reasonable estimation of large pbkp_rediction horizons is hard to obtain, but this may be required by some applications. Over the last years, some researchers have focused on the use of ensembles and meta-learning as a strategy for improving pbkp_rediction accuracy. This paper addresses the problem of selecting and combining models for the design of efficient long-term pbkp_redictors of chaotic time series based on meta-learning and self-organization. We propose and evaluate the use of four heuristic rules for selecting models using a self-organizing map (SOM) neural network and meta-features. The meta-features are extracted from the performances of each involved model when applied to the training time series. A trained SOM map, which was generated using these meta-features, allows the selection of models with diverse behaviors. Two strategies for the combination of models are compared; one is based on the average and a second is based on the median of the forecasts of the selected models. The experiments were executed using four types of series: the time series dataset provided by the NN5 tournament and time series generated from the Mackey-Glass equation, from an ARIMA model and from a sine function. In most cases, the best results were obtained using a percentage of the models belonging to the group that contained the best model. Our results also showed that a combination using a median strategy obtained better results that using an average strategy.
Año de publicación:
2014
Keywords:
Fuente:
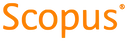
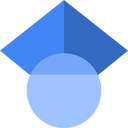
Tipo de documento:
Conference Object
Estado:
Acceso restringido
Áreas de conocimiento:
- Análisis de datos
- Optimización matemática
- Serie temporal
Áreas temáticas:
- Probabilidades y matemática aplicada
- Métodos informáticos especiales
- Sistemas