Selection of Classifiers for Hand Gesture Recognition Using Analytic Hierarchy Process: A Systematic Literature Review
Abstract:
This article presents a systematic study for the selection of classifiers for hand gesture recognition by electromyography signals. The selection of a classifier can be determined using an arbitrary search criterion or employing an Analytic Hierarchy Process (AHP). The classifiers are determined as alternatives, which for the study are K-Nearest Neighbors (KNN), Naïve Bayes (NB), Support Vector Machines (SVM), and Linear Discriminant Analysis (LDA). Each of these alternatives is selected through search criteria, which are average training accuracy, average testing accuracy, sampling fee, average acceptance rating, and scrap rating average. These criteria and alternatives allow through a systematic study and the AHP model to select each search factor of the analysis of the systematic mapping (SMS) quantitatively. The results determine the KNN and SVM classifiers as the most used for research projects in electromyography signal recognition (EMG).
Año de publicación:
2021
Keywords:
- Hand gesture recognition
- Analytic hierarchy process
- EMG
- Systematic Review
Fuente:
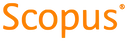
Tipo de documento:
Conference Object
Estado:
Acceso restringido
Áreas de conocimiento:
- Aprendizaje automático
- Ciencias de la computación
Áreas temáticas:
- Ciencias de la computación