Self-adaptive 2D-3D ensemble of fully convolutional networks for medical image segmentation
Abstract:
Segmentation is a critical step in medical image analysis. Fully Convolutional Networks (FCNs) have emerged as powerful segmentation models achieving state-of-the art results in various medical image datasets. Network architectures are usually designed manually for a specific segmentation task so applying them to other medical datasets requires extensive expertise and time. Moreover, the segmentation requires handling large volumetric data that results in big and complex architectures. Recently, methods that automatically design neural networks for medical image segmentation have been presented; however, most approaches either do not fully consider volumetric information or do not optimize the size of the network. In this paper, we propose a novel self-adaptive 2D-3D ensemble of FCNs for 3D medical image segmentation that incorporates volumetric information and optimizes both the models performance and size. The model is composed of an ensemble of a 2D FCN that extracts intra-slice and long-range information, and a 3D FCN that exploits inter-slice information. The architectures of the 2D and 3D FCNs are automatically adapted to a medical image dataset using a multiobjective evolutionary based algorithm that minimizes both the expected segmentation error and number of parameters in the network. The proposed 2D-3D FCN ensemble was tested on the task of prostate segmentation on the image dataset from the PROMISE12 Grand Challenge. The resulting network is ranked in the top 10 submissions, surpassing the performance of other automatically-designed architectures while having 13.3× fewer parameters.
Año de publicación:
2020
Keywords:
- Multiobjective optimization
- deep learning
- Neural Architecture Search
- Medical image segmentation
- Hyperparameter optimization
Fuente:
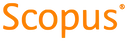
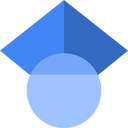
Tipo de documento:
Conference Object
Estado:
Acceso restringido
Áreas de conocimiento:
- Aprendizaje automático
- Laboratorio médico
- Ciencias de la computación
Áreas temáticas:
- Medicina y salud
- Enfermedades
- Ciencias de la computación