Seq2Seq models for recommending short text conversations
Abstract:
The massive amounts of data on social media networks can be overwhelming for users; for this reason, recommending relevant content becomes an essential task to avoid information overload. In this paper, we propose a new task for recommending users that might be interested in join conversations on specific domains. To that end, we introduce a new corpus that contains conversations threads from popular users on Twitter on domains such as politics, sports, or humanitarian activism. Modeling short-text conversations on microblogs can be difficult because user-generated data is unstructured and noisy. Previous works focused on recommending content to users based on latent factors models and collaborative filtering methods. We propose a state-of-the-art recommendation model based on a sequence-to-sequence neural architecture that encodes the text of users’ profiles and the conversations’ context using several variants of recurrent neural networks. The experimental results show that our method provides as much as 20% higher recall compared to baseline methods. Moreover, we use an end-to-end learning framework that allows downstream applications to use recommender systems (RSs) that generalize better to new content by using pre-trained embeddings, thus being useful across domains or events.
Año de publicación:
2020
Keywords:
- recommender systems
- NLP
- Microblogs
- Neural Learning
Fuente:
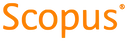
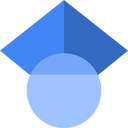
Tipo de documento:
Article
Estado:
Acceso restringido
Áreas de conocimiento:
- Aprendizaje automático
- Ciencias de la computación
- Ciencias de la computación
Áreas temáticas:
- Programación informática, programas, datos, seguridad
- Filosofía de Francia
- Lengua