Sequential pbkp_rediction of quantitative health risk assessment for the fine particulate matter in an underground facility using deep recurrent neural networks
Abstract:
Particulate matter with aerodynamic diameter less than 2.5 µm (PM2.5) in indoor public spaces such as subway stations, has represented a major public health concern; however, forecasting future sequences of quantitative health risk is an effective method for protecting commuters’ health, and an important tool for developing early warning systems. Despite the existence of several pbkp_redicting methods, some tend to fail to forecast long-term dependencies in an effective way. This paper aims to implement a multiple sequences pbkp_rediction of a comprehensive indoor air quality index (CIAI) traced by indoor PM2.5, utilizing different structures of recurrent neural networks (RNN). A standard RNN (SRNN), long short-term memory (LSTM) and a gated recurrent unit (GRU) structures were implemented due to their capability of managing sequential, and time-dependent data. Hourly indoor PM2.5 concentration data collected in the D-subway station, South Korea, were utilized for the validation of the proposed method. For the selection of the most suitable pbkp_redictive model (i.e. SRNN, LSTM, GRU), a point-by-point pbkp_rediction on the PM2.5 was conducted, demonstrating that the GRU structure outperforms the other RNN structures (RMSE = 21.04 µg/m3, MAPE = 32.92%, R2 = 0.65). Then, this model is utilized to sequentially pbkp_redict the concentration and quantify the health risk (i.e. CIAI) at different time lags. For a 6-h time lag, the proposed model exhibited the best performance metric (RMSE = 29.73 µg/m3, MAPE = 29.52%). Additionally, for the rest of the time lags including 12, 18 and 24 h, achieved an acceptable performance (MAPE = 29–37%).
Año de publicación:
2019
Keywords:
- Pbkp_redictive model
- fine particulate matter
- Sequential pbkp_rediction
- Health Risk Assessment
- Recurrent neural networks
Fuente:
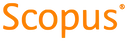
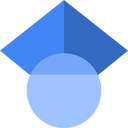
Tipo de documento:
Article
Estado:
Acceso restringido
Áreas de conocimiento:
- Aprendizaje automático
- Ciencias de la computación
Áreas temáticas:
- Métodos informáticos especiales
- Química física
- Física aplicada