Short-Term Load Forecasting in the Distribution System of the Electric Company of Ambato (EEASA) Based on Big Data Criteria
Abstract:
This paper presents a process of obtaining a forecast of the demand for electricity in the Empresa Eléctrica Ambato Regional Centro Norte S.A. (EEASA) using Big Data criteria. A computational model in programming language PYTHON 3.7 was developed. Implementing an electric load projection application in which machine learning algorithms are integrated, such as Autoregressive Integrated Moving Average, Linear Regression, Support Vector Machine and the Multilayer Perceptron. Additionally, different study cases were considered as the most frequent scenarios for the generation of electric load projections such as: a week with holidays, a week with scheduled and unscheduled incidence, a week in dry season and week in rainy season, which allowed test each implemented algorithm, evaluate its results and compare them with each other by calculating the indicators of the percentage of average absolute error (PEMA) and the accuracy of the projection (EP).
Año de publicación:
2019
Keywords:
- Regresion Lineal
- Empresa Eléctrica Ambato
- BIG DATA
- modelo autorregresivo integrado de media móvil
- Machine learning
- proyección de carga eléctrica
- perceptrón multicapa
- máquina de vector soporte
Fuente:
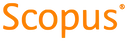
Tipo de documento:
Conference Object
Estado:
Acceso restringido
Áreas de conocimiento:
- Potencia eléctrica
- Big data
- Política energética
Áreas temáticas:
- Ciencias de la computación
- Física aplicada
- Métodos informáticos especiales