Short-term wind speed forecasting over complex terrain using linear regression models and multivariable LSTM and NARX networks in the Andes Mountains, Ecuador
Abstract:
Wind speed forecasting systems over complex terrain at high altitude are very complex and conventional forecasting systems are unable to be applied due to wind variability. This study proposes an approach developed specifically for this study with application of linear regression models as baseline, and Recurrent Neural Networks (RNN): Long Short Term Memory (LSTM) network, and Dynamic Neural Networks (DNN): Nonlinear Autoregressive Exogenous (NARX) network to perform accurate wind speed forecasting in complex terrain in the Ecuadorian Andes to identify feasible places for wind energy applications. This work starts with the installation of two meteorological stations within of the mountainous zone of study to collect measured variables during 2018. Later on, the measured variables were evaluated by using statistical tools and Pearson Correlation Coefficient (PCC) to determine the input variables. Finally, the proposed forecasting models were trained, validated, and tested by using measured data. The DNN and RNN models were compared to determine the best performance through the statistical error forecast measurements as follows, Mean Absolute Percentage Error (MAPE), Mean Squared Error (MSE), and correlation coefficient. The comparison results of the proposed models indicated that the most precise values were for multivariable LSTM network, suggesting this model as a powerful approach to forecast wind speed over complex terrain, demonstrating the importance by using measured variables. Furthermore, the forecasted wind speed showed high values which are suitable for high wind power generation.
Año de publicación:
2022
Keywords:
- Wind speed forecasting
- Ecuadorian andes
- Dynamic Neural Networks
- LSTM network
- NARX network
Fuente:
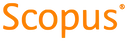
Tipo de documento:
Article
Estado:
Acceso restringido
Áreas de conocimiento:
- Energía
- Meteorología
- Aprendizaje automático
Áreas temáticas de Dewey:
- Otras ramas de la ingeniería
- Física aplicada
- Métodos informáticos especiales

Objetivos de Desarrollo Sostenible:
- ODS 7: Energía asequible y no contaminante
- ODS 13: Acción por el clima
- ODS 9: Industria, innovación e infraestructura
